Just learned from Guy Ernest, that Amazon AWS is setting up a new grant program with focus on Machine Learning. The nearest deadline for submission is January 10st. Don't forget to mention I sent you!
From their call:
"AWS in Education is proud to support selected research projects in machine learning and big data analysis with grants that offer free access to AWS infrastructure services. We are particularly interested in supporting novel applications in the area of distributed data transformation, feature discovery and feature selection, large-scale and/or online classification, regression, recommendation and clustering learning as well as structure discovery. "
Tuesday, December 24, 2013
Friday, December 20, 2013
5th Workshop on Graph Data Management (GDM) - April 4, 2014
I got this from Sherif Sakr (NICTA):
Recently, there has been a lot of interest in the application of graphs in different domains. They have been widely used for data modeling of different application domains such as chemical compounds, multimedia databases, protein networks, social networks and semantic web. With the continued emergence and increase of massive and complex structural graph data, a graph database that efficiently supports elementary data management mechanisms is crucially required to effectively understand and utilize any collection of graphs.
The overall goal of the workshop is to bring people from different fields together, exchange research ideas and results, and encourage discussion about how to provide efficient graph data management techniques in different application domains and to understand the research challenges of such area.
- Paper submission deadline: November 25, 2013 (Extended)
- Author Notification: December 25, 2013
- Final Camera-ready Copy Deadline: January 5, 2014
- Workshop: April 4, 2014
Wednesday, December 18, 2013
WWW 2014 Workshop on Big Graph Mining Announced
BGM is a full-day workshop organized in conjunction with the
23rd International World Wide Web Conference (WWW) in Seoul, Korea on April 7.
Paper submission deadline is January 7, 2014.
http://poloclub.gatech.edu/ bgm2014/
* Organizers
U Kang, KAIST
Leman Akoglu, Stony Brook University
Polo Chau, Georgia Tech
Christos Faloutsos, Carnegie Mellon University
* Workshop Goals
We aim to bring together researchers and practitioners to address
various aspects of graph mining in this new era of big data, such as new
graph mining platforms, theories that drive new graph mining techniques,
scalable algorithms and visual analytics tools that spot patterns and anomalies,
applications that
touch our daily lives, and more. Together, we explore and discuss how
these important facets of are advancing in this age of big graphs.
* Topics of Interest include, but are not limited to
- Scalable graph mining, e.g., parallelized, distributed
- Heterogenous graph analysis
- Complex network analysis
- Graph mining platforms, libraries, and databases
- Interactive/human-in-the-loop graph mining
- Online graph mining algorithms
- Visual analytics and visualization of large graphs
- Analysis of streaming/dynamic/time- evolving graphs
- Machine learning on graphs
- Community detection
- Graph sampling
- Spectral graph analysis
- Social network analysis
- Biological network analysis
- Anomaly detection in graphs
- Active learning / mining
- Theoretical/complexity analysis of graph mining
- Demonstrations of graph mining applications
- Applications of graph mining methods on real-world problems
* Important Dates
* Submission Information
We welcome many kinds of papers such as novel research papers, demo
papers, work-in-progress papers, and visionary (white) papers.
All papers will be peer reviewed, single-blinded.
Authors should clearly indicate in their abstracts the kinds of
submissions that the papers belong to, to help reviewers better
understand their contributions.
Submissions should be in PDF, written in English, with a maximum of 6 pages.
Shorter papers are welcome.
Format your paper using the standard double-column ACM Proceedings Style
http://www.acm.org/sigs/ publications/proceedings- templates
Submit at EasyChair:
http://www.easychair.org/ conferences/?conf=bgm14www
At least one author of an accepted paper must attend the workshop to present
the work. Accepted papers will be published through the ACM Digital Library
If you plan to extend your workshop paper submitted to our BGM'14 workshop,
and submit that extended work to future WWW conferences, please note the
following message from the workshop co-chairs:
"Any paper published by the ACM, IEEE, etc. which can be properly cited
constitutes research which must be considered in judging the novelty of
a WWW submission, whether the published paper was in a conference,
journal, or workshop. Therefore, any paper previously published as part
of a WWW workshop must be referenced and extended with new content to
qualify as a new submission to the Research Track at the WWW conference."
* Further information and Contact
Website: http://poloclub.gatech.edu/ bgm2014/
Email: bgm14www (at) gmail.com
23rd International World Wide Web Conference (WWW) in Seoul, Korea on April 7.
Paper submission deadline is January 7, 2014.
http://poloclub.gatech.edu/
* Organizers
U Kang, KAIST
Leman Akoglu, Stony Brook University
Polo Chau, Georgia Tech
Christos Faloutsos, Carnegie Mellon University
* Workshop Goals
We aim to bring together researchers and practitioners to address
various aspects of graph mining in this new era of big data, such as new
graph mining platforms, theories that drive new graph mining techniques,
scalable algorithms and visual analytics tools that spot patterns and anomalies,
applications that
touch our daily lives, and more. Together, we explore and discuss how
these important facets of are advancing in this age of big graphs.
* Topics of Interest include, but are not limited to
- Scalable graph mining, e.g., parallelized, distributed
- Heterogenous graph analysis
- Complex network analysis
- Graph mining platforms, libraries, and databases
- Interactive/human-in-the-loop graph mining
- Online graph mining algorithms
- Visual analytics and visualization of large graphs
- Analysis of streaming/dynamic/time-
- Machine learning on graphs
- Community detection
- Graph sampling
- Spectral graph analysis
- Social network analysis
- Biological network analysis
- Anomaly detection in graphs
- Active learning / mining
- Theoretical/complexity analysis of graph mining
- Demonstrations of graph mining applications
- Applications of graph mining methods on real-world problems
* Important Dates
Submission : Fri, Jan 17, 2014 (23:59 Hawaii Time)
Acceptance : Mon, Feb 3, 2014
Camera-ready : Mon, Feb 10, 2014
Workshop : Mon, Apr 7, 2014
* Submission Information
We welcome many kinds of papers such as novel research papers, demo
papers, work-in-progress papers, and visionary (white) papers.
All papers will be peer reviewed, single-blinded.
Authors should clearly indicate in their abstracts the kinds of
submissions that the papers belong to, to help reviewers better
understand their contributions.
Submissions should be in PDF, written in English, with a maximum of 6 pages.
Shorter papers are welcome.
Format your paper using the standard double-column ACM Proceedings Style
http://www.acm.org/sigs/
Submit at EasyChair:
http://www.easychair.org/
At least one author of an accepted paper must attend the workshop to present
the work. Accepted papers will be published through the ACM Digital Library
If you plan to extend your workshop paper submitted to our BGM'14 workshop,
and submit that extended work to future WWW conferences, please note the
following message from the workshop co-chairs:
"Any paper published by the ACM, IEEE, etc. which can be properly cited
constitutes research which must be considered in judging the novelty of
a WWW submission, whether the published paper was in a conference,
journal, or workshop. Therefore, any paper previously published as part
of a WWW workshop must be referenced and extended with new content to
qualify as a new submission to the Research Track at the WWW conference."
* Further information and Contact
Website: http://poloclub.gatech.edu/
Email: bgm14www (at) gmail.com
Interesting Sentiment Analysis Demo from Stanford
Following my previous blog post on etcml, I accidentally found out the
related demo http://nlp.stanford.edu:8080/sentiment/rntnDemo.html
And here is the abstract:
This website provides a live demo for predicting the sentiment of movie reviews. Most sentiment prediction systems work just by looking at words in isolation, giving positive points for positive words and negative points for negative words and then summing up these points. That way, the order of words is ignored and important information is lost. In contrast, our new deep learning model actually builds up a representation of whole sentences based on the sentence structure. It computes the sentiment based on how words compose the meaning of longer phrases. This way, the model is not as easily fooled as previous models.
Richard Socher, Alex Perelygin, Jean Wu, Jason Chuang, Christopher Manning, Andrew Ng and Christopher Potts Recursive Deep Models for Semantic Compositionality Over a Sentiment Treebank. Conference on Empirical Methods in Natural Language Processing (EMNLP 2013) pdf
related demo http://nlp.stanford.edu:8080/sentiment/rntnDemo.html
And here is the abstract:
This website provides a live demo for predicting the sentiment of movie reviews. Most sentiment prediction systems work just by looking at words in isolation, giving positive points for positive words and negative points for negative words and then summing up these points. That way, the order of words is ignored and important information is lost. In contrast, our new deep learning model actually builds up a representation of whole sentences based on the sentence structure. It computes the sentiment based on how words compose the meaning of longer phrases. This way, the model is not as easily fooled as previous models.
Richard Socher, Alex Perelygin, Jean Wu, Jason Chuang, Christopher Manning, Andrew Ng and Christopher Potts Recursive Deep Models for Semantic Compositionality Over a Sentiment Treebank. Conference on Empirical Methods in Natural Language Processing (EMNLP 2013) pdf
Tuesday, December 17, 2013
Grades 2014 workshop announced
I am honored to participate as a program committee at Grades 2014 - Graph data management and experience workshop to be held joinly with SIGMOD. The workshop will take place on June 22, 2014 in Snowbird resort in Utah, USA.
Open positions for big data research at Georgia Tech
I got this from my colleague Polo Chao:
===== Open Positions for Postdoctoral Fellows and Research Scientists at Georgia Tech =====
Applications are sought for multiple postdoctoral/research scientist positions in the School of Computational Science and Engineering within the College of Computing at Georgia Institute of Technology. The positions are in the broad area of next generation sequencing and high performance computing/bigdata analytics. Successful candidates will have a strong background in the following areas: bioinformatics, next-generation sequencing, string/graph/parallel algorithms, and writing large, complex HPC software. Candidates who have a subset of these skills with strong interest in acquiring the others are encouraged to apply. All positions are funded by NSF/NIH grants, and can be continued for at least three years contingent on satisfactory annual progress. A minimum of two year commitment is required.
Successful candidates will join a vibrant group in bioinformatics and high performance computing that is engaged in interdisciplinary research with multiple collaborators and industrial partners. Georgia Tech offers competitive benefits and retirement programs. Interested candidates should contact Prof. Srinivas Aluru via email toaluru@cc.gatech.edu.
Srinivas Aluru, Professor
School of Computational Science and Engineering
College of Computing
Georgia Institute of Technology
Klaus Advanced Computing Building
266 Ferst Drive, Atlanta GA 30332
Ph: 404-385-1486 Fax: 404-385-7337
Email: aluru@cc.gatech.edu
URL: http://www.cc.gatech.edu/~ saluru
===== Open Positions for Postdoctoral Fellows and Research Scientists at Georgia Tech =====
Applications are sought for multiple postdoctoral/research scientist positions in the School of Computational Science and Engineering within the College of Computing at Georgia Institute of Technology. The positions are in the broad area of next generation sequencing and high performance computing/bigdata analytics. Successful candidates will have a strong background in the following areas: bioinformatics, next-generation sequencing, string/graph/parallel algorithms, and writing large, complex HPC software. Candidates who have a subset of these skills with strong interest in acquiring the others are encouraged to apply. All positions are funded by NSF/NIH grants, and can be continued for at least three years contingent on satisfactory annual progress. A minimum of two year commitment is required.
Successful candidates will join a vibrant group in bioinformatics and high performance computing that is engaged in interdisciplinary research with multiple collaborators and industrial partners. Georgia Tech offers competitive benefits and retirement programs. Interested candidates should contact Prof. Srinivas Aluru via email toaluru@cc.gatech.edu.
Srinivas Aluru, Professor
School of Computational Science and Engineering
College of Computing
Georgia Institute of Technology
Klaus Advanced Computing Building
266 Ferst Drive, Atlanta GA 30332
Ph: 404-385-1486 Fax: 404-385-7337
Email: aluru@cc.gatech.edu
URL: http://www.cc.gatech.edu/~
Richard Socher - etcml
I got this from both my colleague Chris DuBois and my friend Sagie Davidovich
Richard Socher, a PhD student from Stanford, has released an interesting new tool:
Richard Socher, a PhD student from Stanford, has released an interesting new tool:
http://www.etcml.com/ for text classification.
Monday, December 16, 2013
Data Science Salaries
I got the following email from one of my readers, Frank Lo:
Danny - I really like your blog. I'm a data scientist myself and work within machine learning frameworks every day; I'm always perusing to see what others in the space have to say.
I wanted to share a link and see if you had any interest in mentioning it in a blog post:
Data Science Salary Research
I bet your readers are curious trying to figure out ML/data analysis more clearly as a profession, which is why I wanted to mention it to you. What should people with ML/data science skills expect for salaries when they apply their expertise in industry?
Of course I know the topic is a little different from your regular postings, but I thought it's still very relevant to your base of readers.
It seems the above blog post is conservative by applying a huge range of salaries. I suggest to ignore the lower range and focus on the higher range, especially in the bay area.
Tuesday, December 10, 2013
Ervin Peretz - JSMapreduce
Here is an interesting talk I got from Ervin Peretz:
JSMapreduce a wrapper which tries to make map reduce code writing and deployment easier.
JSMapreduce a wrapper which tries to make map reduce code writing and deployment easier.
Sunday, December 8, 2013
Microsoft AdPredictor (Click Through Rate Prediction) is now implemented in GraphLab!
A couple of years ago, we competed in KDD CUP 2012 and won the 4th place. We used Microsoft's AdPredictor as one of three useful models in this competition as described in our paper: Xingxing Wang, Shijie Lin, Dongying Kong, Liheng Xu, Qiang Yan, Siwei Lai, Liang Wu, Guibo Zhu, Heng Gao, Yang Wu, Danny Bickson, Yuanfeng Du, Neng Gong, Chengchun Shu, Shuang Wang, Fei Tan, Jun Zhao, Yuanchun Zhou, Kang Liu. Click-Through Prediction for Sponsored Search Advertising with Hybrid Models. In ACM KDD CUP workshop 2012.
AdPredictor is described in the paper:
Graepel, Thore, et al. "Web-scale bayesian click-through rate prediction for sponsored search advertising in microsoft's bing search engine." Proceedings of the 27th International Conference on Machine Learning (ICML-10). 2010. html
I tried to look for an open source implementation of AdPredictor and did not find even a single source code. Not surprising, considering the fact that several companies are using it in production for predicting ads CTR (click through rate). So I have decided to go for a fun weekend activity for implementing AdPredictor for GraphLab. The code is available here.
In a nutshell, AdPredictor computes a linear regression model with probit link function.
The input to the algorithm are observations of the type
-1 3:1 4:1 6:1 9:1
1 4:1 5:1 18:1 19:1
...
where the first field -1 is the action (did not click) or 1 (clicked). Next there are pairs of binary features.
The output of the algorithm are weights for each feature. When a new ad comes in, we should simply sum up the weights for the matching features. If the weights are smaller than zero then the prediction is -1 and vice versa.
You are welcome to download graphlab from http://graphlab.org and try it out!
Adpredictor takes file in libsvm format. You should prepare a sub folder with the training file and validation (file needs to end with .validate).
You can run adpredictor using the command:
./adpredictor --matrix=folder/ --max_iter=10 --beta=1
As always let me know if you try it out!
AdPredictor is described in the paper:
Graepel, Thore, et al. "Web-scale bayesian click-through rate prediction for sponsored search advertising in microsoft's bing search engine." Proceedings of the 27th International Conference on Machine Learning (ICML-10). 2010. html
I tried to look for an open source implementation of AdPredictor and did not find even a single source code. Not surprising, considering the fact that several companies are using it in production for predicting ads CTR (click through rate). So I have decided to go for a fun weekend activity for implementing AdPredictor for GraphLab. The code is available here.
In a nutshell, AdPredictor computes a linear regression model with probit link function.
The input to the algorithm are observations of the type
-1 3:1 4:1 6:1 9:1
1 4:1 5:1 18:1 19:1
...
where the first field -1 is the action (did not click) or 1 (clicked). Next there are pairs of binary features.
The output of the algorithm are weights for each feature. When a new ad comes in, we should simply sum up the weights for the matching features. If the weights are smaller than zero then the prediction is -1 and vice versa.
You are welcome to download graphlab from http://graphlab.org and try it out!
Adpredictor takes file in libsvm format. You should prepare a sub folder with the training file and validation (file needs to end with .validate).
You can run adpredictor using the command:
./adpredictor --matrix=folder/ --max_iter=10 --beta=1
As always let me know if you try it out!
Amnon Shashua's TED Talk
Amnon is a Professor at the Hebrew University of Jerusalem, his latest startup is one of the companies that really helps the human kind:
Saturday, December 7, 2013
Ben Lorica at the Data Science Summit
I got this from my colleague Susan Romero: Ben Lorica from O'Reilly Media participated at the data science summit. And of course mentions GraphLab as an interesting system to keep track of!
Thanks Ben!!
Thanks Ben!!
Thursday, December 5, 2013
Trifacta raises additional 12M$ to close the gap between people and data
Fresh news from today. If you like to hear more about Trifacta you should attend our 3rd GraphLab conference! Read some previous Trifacta news.
Wednesday, December 4, 2013
Recommender Systems Course from GroupLens
I got the following course link from my colleague Tim Muss. The GroupLens research group (Univ. of Minnesota) have released a coursera course about recommender systems. Michael Konstan and Michael Ekstrand are lecturing. Any reader of my blog which has an elephant memory will recall I wrote about the Lenskit project already 2 years ago where I intreviewed Michael Ekstrand.
Monday, December 2, 2013
Thursday, November 21, 2013
GraphLab Seattle Users Meetup - Video Online!
Thanks so much for Clive Boulton for his great help in organizing and video capturing our event.
Here is the talk video. I will post the slides soon.
Here is the talk video. I will post the slides soon.
Wednesday, November 20, 2013
Big data research positions
I got contacted by Bosch company who are looking to extend their Palo Alto research center, headed by Soundar Srinivasan. They have 4 open positions:
- ETL & Big Data Architect
- Senior Software Engineer – Analytics
- Big Data ETL Developer
- Senior Manager, ML
Tuesday, November 12, 2013
PowerLyra
We got today the following email from Rong Chen, Shanghai Jiao Tong University:
Hi, GraphLab Experts,
I'm from IPADS group, Shanghai Jiao Tong University, China. This email is aimed at a first time disclosure of project PowerLyra, which is a new hybrid graph analytics engine based on GraphLab 2.2 (PowerGraph).
As you can see, natural graphs with skewed distribution raise unique challenges to graph computation and partitioning. Existing graph analytics frameworks usually use a “one size fits all” design that uniformly processes all vertices and result in suboptimal performance for natural graphs, which either suffer from notable load imbalance and high contention for high-degree vertices (e.g., Pregel and GraphLab), or incur high communication cost among vertices even for low-degree vertices (e.g., PowerGraph).
We argued that skewed distribution in natural graphs also calls for differentiated processing of high-degree and low-degree vertices. We then developed PowerLyra, a new graph analytics engine that embraces the best of both worlds of existing frameworks, by dynamically applying different computation and partition strategies for different vertices. PowerLyra uses Pregel/GraphLab like computation models for process low-degree vertices to minimize computation, communication and synchronization overhead, and uses PowerGraph-like computation model for process high-degree vertices to reduce load imbalance and contention. To seamless support all PowerLyra application, PowerLyra further introduces an adaptive unidirectional graph communication.
PowerLyra additionally proposes a new hybrid graph cut algorithm that embraces the best of both worlds in edge-cut and vertex-cut, which adopts edge-cut for low-degree vertices and vertex-cut for high-degree vertices. Theoretical analysis shows that the expected replication factor of random hybrid-cut is always better than both random vertex-cut and edge-cut. For skewed power-law graph, empirical validation shows that random hybrid-cut also decreases the replication factor of current default heuristic vertex-cut (Grid) from 5.76X to 3.59X and from 18.54X to 6.76X for constant 2.2 and 1.8 of synthetic graph respectively. We also develop a new distributed greedy heuristic hybrid-cut algorithm, namely Ginger, inspired by Fennel (a greedy streaming edge-cut algorithm for a single machine). Compared to Gird vertex-cut, Ginger can reduce the replication factor by up to 2.92X (from 2.03X) and 3.11X (from 1.26X) for synthetic and real-world graphs accordingly.
Finally, PowerLyra adopts locality-conscious data layout optimization in graph ingress phase to mitigate poor locality during vertex communication. we argue that a small increase of graph ingress time (less than 10% for power-law graph and 5% for real-world graph) is more worthwhile for an often larger speedup in execution time (usually more than 10% speedup, specially 21% for Twitter follow graph).
Right now, PowerLyra is implemented as an execution engine and graph partitions of GraphLab, and can seamlessly support all GraphLab applications. A detail evaluation on 48-node cluster using three different graph algorithms (PageRank, Approximate Diameter and Connected Components) show that PowerLyra outperforms current synchronous engine with Grid partition of PowerGraph (Jul. 8, 2013. commit:fc3d6c6) by up to 5.53X (from 1.97X) and 3.26X (from 1.49X) for real-world (Twitter, UK-2005, Wiki, LiveJournal and WebGoogle) and synthetic (10-million vertex power-law graph ranging from 1.8 to 2.2) graphs accordingly, due to significantly reduced replication factor, less communication cost and improved load balance.
The website of PowerLyra: http://ipads.se. sjtu.edu.cn/projects/ powerlyra.html
The latest release has ported to GraphLab 2.2 (Oct. 22, 2013. commit:e8022e6), which aims to provide best compatibility with minimum changes to framework (Perhaps, only add a "type" field to vertex_record.). But this version has no locality-conscious graph layout optimisation now. You can check out the branch from IPADS's gitlab server: git clone http://ipads.se.sjtu. edu.cn:1312/opensource/ powerlyra.git
I did not have time to try it out yet, but it definitely looks like an interesting research direction.
Monday, November 11, 2013
Online Machine Learning Course by Alex Smola & Geoff Gordon (CMU)
Lecture videos should be online:
http://alex.smola.org/teaching/cmu2013-10-701x/index.html
The course is targeted for graduate students.
http://alex.smola.org/teaching/cmu2013-10-701x/index.html
The course is targeted for graduate students.
Wednesday, November 6, 2013
Hunch's Taste Graph
Again, I get this interesting link from my collaborator Chris DuBois. A blog post about Hunch graph,
which is a nice example on how graph data can improve recommendations. And got them bought by ebay a couple of years ago.
which is a nice example on how graph data can improve recommendations. And got them bought by ebay a couple of years ago.
Notable presentation: Datapad @ Strata NY+ PyData
Another interesting presentation I got from my collaborator Chris DuBois.
If you don't know Wes Mckinney, Python Pandas creator and the author of the great book "Python for Data Analysis", you must do yourself a favor and buy this book. It is #1 useful tool for any task involving data analytics.
Anyway, not long ago Wes have founded a company called Datapad who is doing something secretive regarding data analytics. Wes gave an interesting Strata talk, where he did not reveal anything about Datapad, but gave a good overview of companies in the data analytics preparation domain.
In PyData NY, a more detailed talk about the shortcomings of Pandas.
And guess what? Wes just agreed to give a Datapad talk at our 3rd GraphLab conference!! I can't wait to learn more about Datapad.
In PyData NY, a more detailed talk about the shortcomings of Pandas.
And guess what? Wes just agreed to give a Datapad talk at our 3rd GraphLab conference!! I can't wait to learn more about Datapad.
Monday, November 4, 2013
The 3rd GraphLab Conference is coming!
We have just started to organize our 3rd user conference on Monday July 21 in SF. This is a very preliminary notice to attract companies and universities who like to be involved. We are planning a mega event this year with around 800-900 data scientists attending, with the topic of graph analytics and large scale machine learning.
The conference is a non-profit event held by GraphLab.org to promote applications of large scale graph analytics in industry. We invite talks from all major state-of-the-art solutions for graph processing, graph databases and large scale data analytics and machine learning. We are looking for sponsors who would like to contribute to the event organization.
Preliminary talks:
- Reynold Xin, co-Founder of Databricks will present Spark
- Wes McKinney, Founder & CEO of DataPad - TBA
- Prof. Carlos Guestrin, Founder & CEO of GraphLab will present GraphLab
- Prof. Vahab Mirrokni from Google's Pregel team - TBA
- Prof. Joe Hellerstein, Founder & CEO of Trifacta - TBA
- Tao Ye, Senior Scientist, Pandora Internet Radio - TBA
- Josh Wills, Director of Data Science at Cloudera - TBA
- We hope to get a talk from Dr. Avery Ching from Facebook about Giraph.
Preliminary program committee:
The second GRADES workshop, to be held on June 22, 2014 at the premier database systems conference ACM SIGMOD/PODS in Snowbird (Utah), attracts database systems architects, graph data management researchers and practitioners to describe and discuss scenarios, experiences and system internals encountered in managing and analyzing large quantities of graph-shaped data. The GRADES workshop is co-sponsoring the third GraphLab Conference.
- Prof. Joe Hellerstein, Founder & CEO Trifacta & Berkeley
- Prof. Carlos Guestrin, CEO GraphLab & UW
- Mr. Michael Draugelis, Chief Data Scientist, Lockheed Martin
- Mr. Eric Bieschke, Chief Scientist & VP Playlist, Pandora Internet Radio
- Mr. Abhijit Bose, VP Data Science, American Express
- Mr. Richard Mallah, Director of Unstructured and Big Data Analytics, Cambridge Semantics
- Mr. Steven Hillion, VP Product, Alpine Data Labs
- Dr. Jim Kim, VP Product, Skytree
- Prof. Josep LluÃs Larriba Pey, Universidad Polytecnica Di Catalunia
The second GRADES workshop, to be held on June 22, 2014 at the premier database systems conference ACM SIGMOD/PODS in Snowbird (Utah), attracts database systems architects, graph data management researchers and practitioners to describe and discuss scenarios, experiences and system internals encountered in managing and analyzing large quantities of graph-shaped data. The GRADES workshop is co-sponsoring the third GraphLab Conference.
Notable presentation: Mark Levy's Recsys talk
I got this from my collaborator Chris DuBois who sent me Mark Levy's Recsys talk. Previously from last.fm, Mark has generously contributed an implementation of the CLiMF algorithm to GraphChi collaborative filtering toolkit.
What's nice about this talk is examines some of the recent data competition and points some flaws in the way they were constructed.
What's nice about this talk is examines some of the recent data competition and points some flaws in the way they were constructed.
Sunday, October 20, 2013
LSRS 2013 - A great success!
I just heard from LSRS 2013 co-organizers Tao Ye (Pandora) and Quan Yuan (Taobao) that LSRS (large scale recommender systems) workshop we organized as part of RecSys 2013 was a great success. More than 130 researchers attended - it was the workshop with the highest attendance.
Below are some images, with credit to Tao Ye:
There where two notable keynotes. The first from Justin Basilico from netflix. The second from Aapo Kyrola from UW about collaborative filtering with GraphChi. The full papers, along with other interesting talks are found here.
Thanks to Pandora for hosting the happy hour just after the workshop:
Below are some images, with credit to Tao Ye:
There where two notable keynotes. The first from Justin Basilico from netflix. The second from Aapo Kyrola from UW about collaborative filtering with GraphChi. The full papers, along with other interesting talks are found here.
Thanks to Pandora for hosting the happy hour just after the workshop:
Stay tuned for next year's workshop - this time in SF!
Friday, October 18, 2013
Total subgraph communicability in GraphLab
Our user Jacob Kesinger has implemented total subgraph communicability in GraphLab v2.2.
By the way, Jacob is looking for a data scientist position in the area between San Jose and Palo Alto. So if you are looking for PhD in math, with a lot of applied knowledge, I truly recommend Jacob!
(And tell him I sent you.. :-) Anyway he is his description of the method:
I'm posting to the list to announce that I've implemented Total
Thanks Jacob for your great contribution!!
By the way, Jacob is looking for a data scientist position in the area between San Jose and Palo Alto. So if you are looking for PhD in math, with a lot of applied knowledge, I truly recommend Jacob!
(And tell him I sent you.. :-) Anyway he is his description of the method:
I'm posting to the list to announce that I've implemented Total
Subgraph Communicability, a new centrality measure due to
Benzi&Klymco[0]. For a directed graph with adjacenty matrix A,
TSC_i = sum_j exp(A)_{ij} = (exp(A)*1)_i.
This code calculates the TSC using an Arnoldi iteration on the Krylov
subspace {b, Ab,A*Ab, A*A*Ab, ...} due to Saad[1], and using the new
warp engine from Graphlab 2.2 (without which this would have been, at
best, very challenging).
I don't have access to a cluster right now, so I can't test that it performs
properly in the distributed environment, but it works fine on a single
macbook air (and can compute TSC for a graph with 130K nodes and 1.4M
edges in less than a minute under load).
Small components of large graphs will have bogus answers due to
floating point issues. To find the exact TSC for a particular node i,
run with "--column i" to find exp(A)*e_i; you will have to sum the
resulting output yourself, however.
SAMPLE INPUT:
0 1
1 2
1 3
2 4
3 4
1 0
2 1
3 1
4 2
4 3
OUTPUT:
0 5.17784
1 10.3319
2 8.49789
3 8.49789
4 7.96807
You can verify this in python as:
import scipy
import scipy.linalg
A = scipy.array([[0,1,0,0,0],[1,0, 1,1,0],[0,1,0,0,1],[0,1,0,0,1] ,[0,0,1,1,0]])
scipy.linalg.expm2(A).sum( axis=1)
The code is here: https://github.com/kesinger/ graphlab
And I've written more about it here: http://jacobkesinger.tumblr. com/post/64338572799/total- subgraph-centrality
[0]: Benzi, Michele, and Christine Klymko. Total Communicability as a Centrality Measure. ArXiv e-print, February 27, 2013.http://arxiv.org/abs/1302.6770 .
[1]: Saad, Yousef. “Analysis of Some Krylov Subspace Approximations to the Matrix Exponential Operator.” SIAM Journal on Numerical Analysis 29, no. 1 (1992): 209–228.
Wednesday, October 16, 2013
Parallel and distributed algorithms for inference and optimization workshop - Oct 21-24 UC Berkeley
My friend and colleague Michael Mahoney is one of the organizers of this workshop: Parallel and distributed algorithms for inference and optimization. The workshop takes place October 21-24 in UC Berkeley.
A notable interesting talk by my friend and collaborator Joey Gonzalez: Scalable graph parallel inference algorithms and systems.
A notable interesting talk by my friend and collaborator Joey Gonzalez: Scalable graph parallel inference algorithms and systems.
Monday, October 14, 2013
How clean are SF restaurants?
I stumbled upon this interesting tutorial with a unique freely available dataset. Tutorial credit: Zipfian Academy
Friday, October 11, 2013
Kaggle Titanic Contest Tutorial
I found this great tutorial written by Andrew Conti, a statistician & consultant from NY. The tutorial is using ipython as an interactive way for teaching data scientists how to better understand the data and perform classification using various tools.
The topic is the Titanic contest I mentioned here earlier.
Here is a snapshot:
What is nice about this tutorial is that it is interactive, you can follow by running each step, changing parameters on the fly.
The topic is the Titanic contest I mentioned here earlier.
Here is a snapshot:
What is nice about this tutorial is that it is interactive, you can follow by running each step, changing parameters on the fly.
Wednesday, October 9, 2013
First Spark Summit
Databricks, the newly founded Spark based company has kindly asked us to share our event organizer, Courtney Burton, for their first Spark Summit.
The event will take place on Monday December 2, 2013 in SF.
Readers of my blog are welcome to use the following discount code.
The event will take place on Monday December 2, 2013 in SF.
Readers of my blog are welcome to use the following discount code.
Monday, October 7, 2013
New SOSP paper: a lightweight infrastructure for graph analytics
I got this reference from my collaborator Aapo Kyorla, author of GraphChi.
A Lightweight Infrastructure for Graph Analytics. Donald Nguyen, Andrew Lenharth, Keshav Pingali (University of Texas at Austin), to appear in SOSP 2013.
It is an interesting paper which heavily compares to GraphLab, PowerGraph (GraphLab v2.1) and
GraphChi.
One of the main claims is that dynamic and asynchronous scheduling can significantly speed up many graph algorithms (vs. bulk synchronous parallel model where all graph nodes are executed on each step).
Some concerns I have is regarding the focus on multicore settings, which makes everything much easier, and thus to comparison with PowerGraph less relevant.
Another relevant paper which improves on GraphLab is: Leveraging Memory Mapping for Fast and Scalable Graph Computation on a PC. Zhiyuan Lin, Duen Horng Chau, and U Kang, IEEE Big Data Workshop: Scalable Machine Learning: Theory and Applications, 2013. The basic idea is to speed graph loading using mmap() operation.
A Lightweight Infrastructure for Graph Analytics. Donald Nguyen, Andrew Lenharth, Keshav Pingali (University of Texas at Austin), to appear in SOSP 2013.
It is an interesting paper which heavily compares to GraphLab, PowerGraph (GraphLab v2.1) and
GraphChi.
One of the main claims is that dynamic and asynchronous scheduling can significantly speed up many graph algorithms (vs. bulk synchronous parallel model where all graph nodes are executed on each step).
Some concerns I have is regarding the focus on multicore settings, which makes everything much easier, and thus to comparison with PowerGraph less relevant.
Another relevant paper which improves on GraphLab is: Leveraging Memory Mapping for Fast and Scalable Graph Computation on a PC. Zhiyuan Lin, Duen Horng Chau, and U Kang, IEEE Big Data Workshop: Scalable Machine Learning: Theory and Applications, 2013. The basic idea is to speed graph loading using mmap() operation.
Sunday, September 29, 2013
Thursday, September 19, 2013
GraphLab Internship Program (Machine Learning Summer Internship)
We are glad to announce our latest internship program for the summer of 2014. We have around 15 open positions, either at GraphLab/UW or affiliated companies we work with.
Would you like to have a chance to deploy cutting edge machine learning algorithms in practice? Do you want to get your hands on the largest and most interesting datasets out there? Do you have valuable applied experience working with machine learning in the cloud? If so, you should consider our internship program.
Candidates must be US-based PhD or master students in one of the following areas: machine learning, statistics, AI, systems, high performance computing, distributed algorithms, or math. We are especially interested in those who have used GraphLab/GraphChi for a research project or have contributed to the GraphLab community.
All interested applicants should send their resume to internships@graphlab.com. If you are a company interested having a GraphLab intern, please feel free to get in touch.
Here is a (very preliminary) list of open positions:
GraphLab is a graph-based, high performance, open source distributed computation framework written in C++. While GraphLab was originally developed for Machine Learning tasks, it has found great success at a broad range of other data-mining tasks; out-performing other abstractions by orders of magnitude. Our latest beta is a cloud based ipython interface to GraphLab. GraphLab office is located in UW campus in Seattle.
Rocket Fuel is a digital-advertising technology company in Silicon Valley that has grown rocket-fast since its founding in 2008. It is the leading provider of artificial intelligence online-advertising solutions. It combines a world-class engineering team with the industry's most productive sales and delivery teams. Its customers are some of the world’s most successful brands. Rocket Fuel serves them from its offices in 19 cities around the globe. Engineering is located in Redwood City (Bay Area), CA
Technicolor is an industry leader in the production of video content for movies, TV, advertising, games and more. The Palo Alto research lab offers internship positions in the fields of machine learning, optimization, networking, and systems, focusing on high quality academic publications. We encourage applications from highly motivated Ph.D. candidates in Computer Science, Electrical and Computer Engineering, Statistics and Applied Math, with a strong background in one of the above fields. Technicolor Labs are located downtown Palo Alto.
CloudCV is a large-scale distributed computer vision algorithm package, running on the cloud. CloudCV is a project developed at the Machine Learning and Perception Lab, Virginia Tech, led by Prof. Dhruv Batra. CloudCV uses GraphLab for implementing distributed computer vision algorithms. Some of the functionality is derived from using OpenCV with Graphlab SDK.
Tagged makes social discovery products that enable anyone to meet and socialize with new people. Our mission is to help everyone feel love and belonging, and we're building toward a vision where anyone can use a device to instantly connect with interesting new people anytime, anywhere. Founded in 2004 and profitable since 2008, Tagged is a market leader in social discovery with over 300 million registered members in 220 countries who make over 100 million new social connections every month.
Rackspace is the leading innovator in cloud computing and one of the
founders and largest contributors to OpenStack. Rackers have the
freedom to do great work and the challenge of using their skill and
ambition to drive the future of the Web. Rackspace Cloud provides
on-demand scalable website, application, and storage hosting. We
enable developers and business decision-makers to avoid the hassles
and costs of dedicated hardware while offering the infinite
scalability of the Cloud.
Rackspace has an inspiring culture which provides room for growth and
innovation. We have multiple public and internal facing tech events
each week. Rackspace is ranked on Forbes Fastest Growing Technology
Companies list and is on Fortune and Glassdoor's Top 100 Companies to
work for.
Learn more about us:
http://developer.rackspace.com/
http://rackertalent.com/sanfrancisco/
http://www.rackspace.com/blog/
Want to work on the science behind the largest scale music service in the US?
Pandora's science team is building the next innovations in machine learning algorithms that help hundreds of millions of listeners discover music they love. We're looking for 3-4 interns (preferably 2nd or 3rd year Ph.D. Students) whose research interests are aligned with ML and are passionate about music. Potential topics include personalization, scalable algorithms, real time and effective recommendation measurement, user modeling, etc. Please apply here: http://www.pandora.com/careers/position?id=o06RXfwE, and indicate in your cover letter that you heard about the position from the GraphLab blog.
StumbleUpon is a user-curated web content discovery engine that recommends relevant, high quality pages and media to its users, based on their interests. More than 30 million people turn to StumbleUpon to be informed, entertained and surprised by content and information recommended just for them. In addition, more than 100,000 brands, publishers and other marketers have used StumbleUpon’s Paid Discovery platform to tell their stories and promote their products and services. StumbleUpon offices are located downtown San Francisco
Intel Labs is addressing the challenges of Big Data Analytics with disruptive technologies and is focused on delivering new software and hardware technologies that will transform datacenter operations and improve user experience. It is driving innovations to better handle data both big and small, from small-scale sensor networks that enable data gathering to large-scale cloud resources for deep analysis. And, it is developing knowledge discovery platforms to support emergent applications, from personalized recommendation systems to targeted drug therapies. We are interested in talking to MS and PhD candidates with excellent programming skills and coursework in machine learning, data mining, or parallel computing systems.
Comcast Labs Washington DC is an innovative research group within Comcast's Metadata Processing and Search Services unit that does groundbreaking research to develop the video and TV search & discovery technologies that support Comcast’s approximately 25 Million subscribers. Our projects focus on Machine Learning for Recommendations, Search and Click-through Prediction, NLP for Voice-based Interfaces, Video Annotation/Segmentation of premium video, and other Big Data problems. Comcast is the largest provider of TV and Broadband Services in North America, the largest provider of TV search and discovery applications in North America, & the 6th largest provider of search on the web.
The Social Computing Group at Adobe is working with data from Behance, a social network for creatives that joined Adobe in January 2013, developing an infrastructure that leverages this social graph to extract value for applications like talent search, recommendations and graph search. It is using Machine Learning and Graph Mining techniques to extract aggregate patterns and help users find work, interesting content and people. Behance is at the core of Adobe push to make the creative community more interconnected, organized and leverage each other's work to be more productive and successful."
LivingSocial is the local marketplace to buy and share the best things to do in your city. With unique and diverse offerings each day, we inspire members to discover everything from weekend excursions to one-of-a-kind events and experiences to exclusive gourmet dinners to family aquarium outings and more. We are interested in interns with in-depth knowledge of machine learning and/or recommendation systems as well as excellent programming skills who would like to help us solve unique marketing problems through leveraging big data infrastructures and tools.

Tapad Inc. is the undisputed leader in cross-platform marketing technology. The company’s groundbreaking technologies address the new and ever-evolving reality of media consumption on smartphones, tablets and home computers. Through Tapad, advertisers are now able to get a unified view of consumers across all screens. Tapad has built the most robust, real-time cross-platform audience buying technology available. Tapad is backed by major venture firms and “a hell of a list of entrepreneurs who created some of the most valuable online advertising companies of the last decade” (TechCrunch). Tapad is based in New York and has offices in Atlanta, Chicago, Dallas, Detroit, Los Angeles, Miami and San Francisco.
CBS Interactive, a division of CBS Corporation, is the premier online content network for information and entertainment. With more than 250 million people visiting its properties each month, it is a top 10 Web property globally and a top 5 Web property in the U.S. in terms of unique video viewers. Its portfolio of leading brands, which include CNET, CBS.com, CBSNews.com, CBSSports.com, GameSpot, TVGuide.com, TV.com and Last.fm, span popular categories like technology, entertainment, sports, news and gaming.

Rdio is the groundbreaking digital music service that is reinventing the way people discover, listen to, and share music. With on-demand access to over 20 million songs, Rdio connects people with music and makes it easy to search for and instantly play any song, album, artist, or playlist. Launched in August 2010, Rdio is headquartered in San Francisco and was founded by Janus Friis, the co-creator of Skype. Available in countries all over the world, Rdio is funded by Janus Friis through his investment entities, Atomico, and Skype.
Stay tuned - additional companies will be added soon.
ExxonMobil’s Corporate Strategic Research is currently offering graduate-level internships for summer of 2014 in the area of large scale machine learning within our Data Analytics and Optimization Section. During the three month internship, working closely with researchers in our team, the candidate will be expected to refine the problem definition, conduct fundamental research on algorithms and theory, demonstrate results on a prototype application and
prepare material for publication. The mission of Corporate Strategic Research is unique within the ExxonMobil Corporation. We are tasked with creating science-based opportunity and competitive advantage for ExxonMobil by conducting high-risk, high-reward research aligned with the Corporation’s business objectives. The laboratory is located 50 miles from New York City in scenic western New Jersey. The
successful candidate will join a dynamic group of scientists performing breakthrough research for all sectors of the corporation, developing new approaches to solve our most challenging problems. ExxonMobil offers an excellent working environment and competitive compensation. Start and end dates are flexible, typically encompassing mid-May to late-August. Subsidized housing
is offered.
The Bosch Research and Technology Center, with labs in Palo Alto, CA, Pittsburgh, PA, and Cambridge, MA focuses on innovative research and development for the next generation of Bosch products. The data mining group creates new data mining and large-scale machine learning algorithms for high-performance, distributed and parallel computing environments. Our problems deal with sparse, high-dimensional heterogeneous data that have temporal correlations, missing values and asynchronous streams. Topics that we work on include time-series analysis, latent variable models, sparse and missing data problems, association rule mining, to name a few. Our models and methods are implemented in a distributed, parallelized architecture and run on our HPC cluster in order to scale up to Big data sets. Internships are expected to be at least 10-12 weeks long during the summer months. Previous internships in our group have led to successful publications and/or patents. More information can bout found at http://www.bosch.us/content/language1/html/9799.htm
Would you like to have a chance to deploy cutting edge machine learning algorithms in practice? Do you want to get your hands on the largest and most interesting datasets out there? Do you have valuable applied experience working with machine learning in the cloud? If so, you should consider our internship program.
Candidates must be US-based PhD or master students in one of the following areas: machine learning, statistics, AI, systems, high performance computing, distributed algorithms, or math. We are especially interested in those who have used GraphLab/GraphChi for a research project or have contributed to the GraphLab community.
All interested applicants should send their resume to internships@graphlab.com. If you are a company interested having a GraphLab intern, please feel free to get in touch.
Here is a (very preliminary) list of open positions:
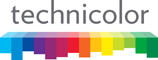
http://rackertalent.com/sanfrancisco/
http://www.rackspace.com/blog/
Zillow, Inc. operates the largest home-related marketplaces on mobile and the Web, with a complementary portfolio of brands and products that help people find vital information about homes, and connect with the best local professionals. In addition, Zillow operates an industry-leading economics and analytics bureau led by Zillow's Chief Economist Dr. Stan Humphries. Dr. Humphries and his team of economists and data analysts produce extensive housing data and research covering more than 350 markets at Zillow Real Estate Research.
Pandora's science team is building the next innovations in machine learning algorithms that help hundreds of millions of listeners discover music they love. We're looking for 3-4 interns (preferably 2nd or 3rd year Ph.D. Students) whose research interests are aligned with ML and are passionate about music. Potential topics include personalization, scalable algorithms, real time and effective recommendation measurement, user modeling, etc. Please apply here: http://www.pandora.com/careers/position?id=o06RXfwE, and indicate in your cover letter that you heard about the position from the GraphLab blog.

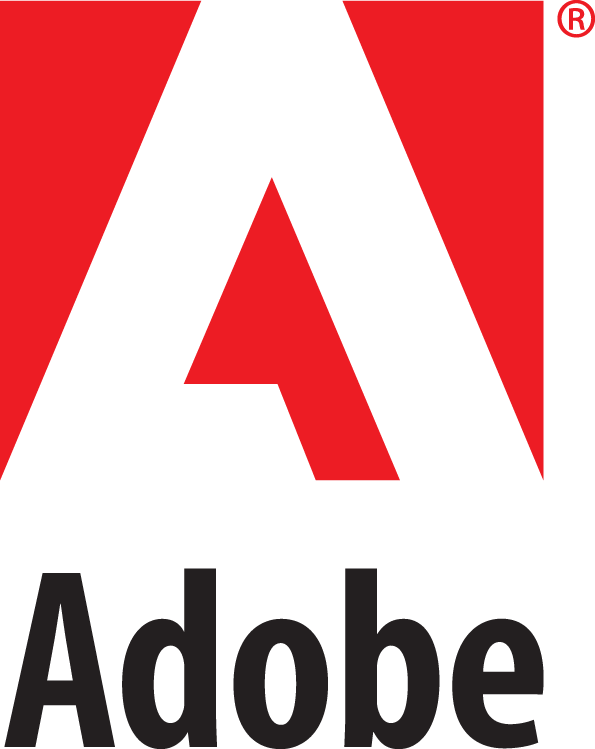
LivingSocial is the local marketplace to buy and share the best things to do in your city. With unique and diverse offerings each day, we inspire members to discover everything from weekend excursions to one-of-a-kind events and experiences to exclusive gourmet dinners to family aquarium outings and more. We are interested in interns with in-depth knowledge of machine learning and/or recommendation systems as well as excellent programming skills who would like to help us solve unique marketing problems through leveraging big data infrastructures and tools.

Tapad Inc. is the undisputed leader in cross-platform marketing technology. The company’s groundbreaking technologies address the new and ever-evolving reality of media consumption on smartphones, tablets and home computers. Through Tapad, advertisers are now able to get a unified view of consumers across all screens. Tapad has built the most robust, real-time cross-platform audience buying technology available. Tapad is backed by major venture firms and “a hell of a list of entrepreneurs who created some of the most valuable online advertising companies of the last decade” (TechCrunch). Tapad is based in New York and has offices in Atlanta, Chicago, Dallas, Detroit, Los Angeles, Miami and San Francisco.
Rdio is the groundbreaking digital music service that is reinventing the way people discover, listen to, and share music. With on-demand access to over 20 million songs, Rdio connects people with music and makes it easy to search for and instantly play any song, album, artist, or playlist. Launched in August 2010, Rdio is headquartered in San Francisco and was founded by Janus Friis, the co-creator of Skype. Available in countries all over the world, Rdio is funded by Janus Friis through his investment entities, Atomico, and Skype.
Stay tuned - additional companies will be added soon.
prepare material for publication. The mission of Corporate Strategic Research is unique within the ExxonMobil Corporation. We are tasked with creating science-based opportunity and competitive advantage for ExxonMobil by conducting high-risk, high-reward research aligned with the Corporation’s business objectives. The laboratory is located 50 miles from New York City in scenic western New Jersey. The
successful candidate will join a dynamic group of scientists performing breakthrough research for all sectors of the corporation, developing new approaches to solve our most challenging problems. ExxonMobil offers an excellent working environment and competitive compensation. Start and end dates are flexible, typically encompassing mid-May to late-August. Subsidized housing
is offered.
First Seattle GraphLab Users Meetup - Thursday November 21 in Seattle
Will take place on Thursday November 21 at the GraphLab office (in UW campus) in Seattle.
We will formally release our GraphLab Notebook (beta). Everyone is invited!
Place is limited. Please RSVP here.
We will formally release our GraphLab Notebook (beta). Everyone is invited!
Place is limited. Please RSVP here.
Saturday, September 14, 2013
Predicting personal information from Facebook likes data
I got from Carlos del Cacho, a GraphLab user and a data scientist from Traity, a link to the following interesting paper:
Private traits and attributes are predictable from digital records of human behavior, by Michal Kosinskia, David Stillwella and Thore Graepelb, in PNAS 2013.
While this result is not surprising, and the method used is rather basic, it is still a nice demonstration of a fact we are all aware of, that machine learning is a powerful tool in predicting various user properties.
The authors use the following simple construction: create a user vs. likes matrix, decompose it using SVD with 100 dimensions, and then perform linear regression for each field of interest to find weights for each singular vector. Once a new user is observed, those weights are produced to compute prediction. (Logistic regression was used for binary categorical variables).
And here are some of the results. Each number signifies the success in prediction.
Private traits and attributes are predictable from digital records of human behavior, by Michal Kosinskia, David Stillwella and Thore Graepelb, in PNAS 2013.
While this result is not surprising, and the method used is rather basic, it is still a nice demonstration of a fact we are all aware of, that machine learning is a powerful tool in predicting various user properties.
The authors use the following simple construction: create a user vs. likes matrix, decompose it using SVD with 100 dimensions, and then perform linear regression for each field of interest to find weights for each singular vector. Once a new user is observed, those weights are produced to compute prediction. (Logistic regression was used for binary categorical variables).
And here are some of the results. Each number signifies the success in prediction.
Another related paper which comes into mind is the paper by my friend Udi Weinsberg from Technicolor Labs:
"BlurMe: Inferring and Obfuscating User Gender Based on Ratings," Udi Weinsberg, Smriti Bhagat, Stratis Ioannidis and Nina Taft. ACM Conference on Recommender Systems (RecSys), 2012.
Wednesday, September 11, 2013
ParLearning 2014 workshop
The workshop will be held on May 23, 2014 in Pheonix AZ. Workshop highlights are:
* Foster collaboration between HPC community and AI community
* Applying HPC techniques for learning problems
* Identifying HPC challenges from learning and inference
* Explore a critical emerging area with strong academia and industry interest
* Great opportunity for researchers worldwide for collaborating with Academia and Industry
Submission date is December 30, 2013.